Artificial Intelligence: The New Driving Force Behind Sports Performance and Entertainment
13 February 2019
If I was to raise the topic of “artificial intelligence” in football, you’d be forgiven for thinking I was referring to Joey Barton’s decision to enrol as a philosophy student at Roehampton University back in 2013. But not so – last month it was announced that London-based non-league club, Wingate & Finchley FC, have employed football’s very first ‘AI coach’.
Developed by Greenshoot Labs, the voice-led technology will build an in-depth knowledge of the team’s individual attributes. It will select the team’s starting XI, formation and tactics, and recommend bespoke match strategies and substitutions to counter upcoming opponents in the Isthmian Premier League. Through machine learning, the software will develop, improve and adapt its tactics over time the more data it gathers (a function Jose Mourinho has, evidently, yet to be installed with). Wingate’s first outing since the new addition to its backroom staff ended 1-1.
Technology once confined to the Championship Manager video game series has now entered the real-world coaching fold – a digital outsider here to shake things up in the muddy, analogue environment of non-league football. But this particular breakthrough should be seen only as the next step in a sporting revolution that is both well underway, and only in its infancy. AI has permeated many parts of the sports industry already and will continue to do so – raising interesting questions around the need for AI-specific regulations and ultimate ‘ownership’ of the vast swathes of data these technologies collect.
Emergence of AI in Sport
Artificial intelligence is a broad concept. At its core, it involves designing “intelligent” machines that, like humans, are capable of acquiring and employing knowledge, information and professional skills for the overall purpose of problem solving. It encompasses data analytics, predictive analysis, machine learning, pattern recognition and, at the very least, a simulation of sentience (we’ll leave existential questions of consciousness parked for another day).
Data analytics is by no means new to sport. “Moneyball” – the story of Billy Beane’s sabermetric approach to selection and management of the Oakland A’s baseball team during the early 2000s – is perhaps one of the best known early uses of analytics by a sports team.
But since then, we’ve witnessed a quantum leap in machine learning, AI and data science. The development of wearable technology and RFID tags means we are now able to monitor an athlete’s movement and health data like never before. Equally, advances in computer vision have made it possible for computers to gather increasingly more information from every second of a video feed. As the technology behind AI has grown, so has its wider use in professional sport.
.jpg?la=en&hash=B8D520EC41B402CEEC2B1065C4D5E13427E88F5C)
A Tool for Success
Much of the “intelligence” that underpins today’s leading sports AI software revolves around big data and pattern recognition, combining to give sports teams a competitive edge.
Rajiv Maheswaran, CEO and Co-Founder of Second Spectrum, has spoken about the AI technology his team built which has transformed the way player movement in basketball can be tracked and analysed. The machine intelligence works by collecting mass amounts of raw data on player movements and tactics (“spacio-temporal features”) - these range from the fundamentally simple (e.g. tracking a player’s movement throughout a game) to the more complex (e.g. assessing a player’s propensity to successfully deploy a “pick and roll” manoeuvre in a particular position on the court).
From here, Second Spectrum is able to identify patterns and variations with such precision and recall, that it can determine the likelihood of a player shooting; the quality of the shot; the likelihood of shot success; the likelihood of a rebound (etc.), all the while factoring in differentials such as whether it’s a good shooter taking a bad shot, or a bad shooter taking a good shot (etc.). Almost every NBA team now utilises this software in an effort to win more games.
Away from basketball, data juggernauts like Sportlogiq, Opta and Genius Sports are leading the way in sports analytics and data capture. Sportlogiq in particular – established by former Canadian Olympic pair skater Craig Buntin – has developed an AI platform in partnership with a number of teams in the National Hockey League (NHL). The AI monitors over 158 million data points per NHL game on a latency of milliseconds, to provide insights as deep as to what extent a pass was made under pressure or whether that was the optimal pass in the situation. Crucially, the AI product automates the process of data capture, removing the human element by which most sports data has been collected up to now.
With enhanced algorithms crunching more data than ever before, sports teams are developing a deeper understanding of the factors that can determine results on the pitch. This has led to the emergence of new metrics by which to analyse team performance, none more ‘en vogue’ than Opta’s “xG” (expected goals), which quantitatively measures chance quality in football. By analysing the historical data from over 300,000 shots and factoring in the variations that determine chance quality (who was taking the shot, proximity and angle to goal, time and space to shoot etc.), xG calculates how likely it is that a particular shot will be scored (once in every 10 would be worth 0.10 xG). An xG model, made possible by big data, goes beyond simply looking at “number of shots/shots on target” and is even a more a consistent measure of overall performance than actual goals scored (although scoring actual goals should remain the number one priority).
Wearable Tech
AI affords individual athletes the opportunity to improve their skills, physical preparedness and mental conditioning. Wearables such as Catapult and Zephyr are now commonplace on the training ground. These devices directly measure key physiological inputs including heart rate, breathing rate, heart rate variability, posture and impact, which can then be used to deduce secondary parameters such as jump height, explosiveness, peak force and heartrate recovery. With the assistance of accompanying AI applications that break-down and communicate the raw data back to the athlete, the statistics gathered allow athletes to assemble a ‘quantified self’. This rich and hugely personalised dataset can be used to optimise an athlete’s training schedule for improved performance.
Player Recruitment
Statistical data now plays a key role in player recruitment. Whether it be in identifying young talent that have a high probability of excelling in future, or spotting players that are undervalued by the market, AI algorithms help coaches and scouts ensure talented players don’t escape notice.
Baseball scouts in the US are a prime example. Long-time users of statistics to scout and evaluate players, teams are now beginning to use AI to analyse an ever-expanding set of player data — this includes radar gun data (measuring throwing speed and spin), video tracking (how players move around the field or adopt a particular batting posture) and swing speed mechanics from sensor-studded bats.
Whilst for some there will never be a replacement for the good old-fashioned ‘easy on the eye’ test when it comes to scouting, detailed player data collected by AI gives today’s scouts a statistical consideration that cannot be ignored.
Adjudication
AI is able to adjudicate the minutiae and make tough calls the human eye can’t always catch.
Hawk-Eye technology offers a consistent and valuable second opinion on the accuracy of line-calls in tennis (reducing McEnroe-style meltdowns in the process). That same Hawk-Eye technology also widely powers goal-line technology (GLT), introduced into competitive football at the FIFA Club World Cup in December 2012 (2½ years too late for England fans still reeling from Frank Lampard’s “goal that never was” against Germany at the 2010 World Cup). GLT works by using a network of high-speed video cameras to track a ball’s position at a given time via triangulation. Hawk-Eye can tell when the (whole of the) ball has crossed the goal-line, and the software then alerts the match officials via radio transmission to the referee’s watch. And thus, the “it was over the line / oh no it wasn’t” pantomime of days gone by has been quashed.
In the latest development in this area, gymnastics fans will be salivating to hear that the International Gymnastics Federation (FIG) plans to use AI to help judges score the 2020 Olympic Games in Tokyo. The AI system (developed by Japanese IT experts, Fujitsu) processes data collected via 3D sensors during gymnasts’ performances and provides instant feedback about each performance, from the height of an athletes’ vault to the angle of their legs during a balance beam routine. The technology will help to counteract any inherent subjectivity or unconscious bias amongst the judging panel.
Putting the “AI” into Sporting Entertainment
AI represents a game-changer in the sports entertainment industry for the benefit of viewers, broadcasters, advertisers and sponsors alike.
From a broadcasting perspective, the increased capture of raw data means that video content can be enriched with more innovative statistical insights. AI also now serves a role in the production process for live sport – helping producers and editors do their job faster and more efficiently. Based on deep learning methods, AI can support directors by automating certain tasks, analysing video and preparing A/V assets to be shown in real-time, whether that be in the form of replays, highlights, or a compelling selection of camera angles. For the 2018 Wimbledon tennis championships, IBM successfully taught the Watson supercomputer to better recognize player emotions and crowd noise to help screen through video clips and identify key moments of the tennis tournament, which it was then able to serve up via its AI highlights solution.
Brand-tracking AI solutions like VBrand and IdenTV are changing the way brands are able to measure their video marketing efforts. Powered by computer vision, automated speech recognition and textual semantic classifiers (amongst other smart functions), image recognition technology can capture how often a brand was seen on television screens; how long it was on display for; and the quality of exposure. This allows sports sponsors to build a ‘big data’ picture about their campaigns – e.g. quantifying how many times a brand logo appears over a season’s worth of TV, online and across other mediums.
Machine learning and AI are enabling rights holders and advertisers to offer innovative solutions and value to customers in new ways. Fans watching the same live stream or video upload can be served different, tailored ads based on their personal preference and behaviour – this the result of advanced machine learning algorithms making ad servers smarter than ever before.
AI also operates to improve and streamline the experience for fans in-stadia. Facial recognition technologies have developed to a point where they are now able to identify people moving at full speed, helping event hosts manage the access of fans within the stadium, speed up purchase processes, and spot known trouble makers and security threats. And let us not overlook the prevalence of AI “chatbots”, which frequently help today’s clubs and event organizers communicate personally with fans (AS Roma unveiled its new ‘Botistuta’ chatbot in November 2018, a prudent signing which will bolster its customer service frontline).
How is AI regulated in the UK?
As it stands, there is an absence of concrete regulation governing the deployment of AI in the UK. The House of Lords Select Committee on Artificial Intelligence reported in April 2018 that there is no immediate need for a comprehensive set of AI-specific regulations, which leaves the domestic AI environment fairly developer-friendly for now. The report recommended that it should be the responsibility of the (newly established) Government Office for AI, working in tandem with the Centre for Data Ethics and Innovation, to consider whether there is a pressing need to introduce AI-specific regulations in future.
Data Privacy
The main regulatory touchpoint for AI at this moment in time is data protection and privacy law. As AI solutions invariably log vast amounts of data in order to operate, in many cases this involves processing certain quantities of personal data, which can be used to build-up an identifiable profile of a particular individual.
The GDPR (as complemented by the Data Protection Act 2018) regulates the use and processing of personal data in the UK. Under Article 22, in absence of specific circumstances (such as where the individual has given their explicit consent), data subjects have a right not to be subject to decisions based solely on automated processing (including profiling) that produce legal effects concerning the individual. Returning to our AI football coach example, it is conceivable that the algorithm could continually recommend leaving a particular player on the bench on the basis of his statistics, much to the detriment of his career. As such, football clubs like Wingate & Finchley FC would be wise to demonstrate that there has been an appropriate degree of human-based decision making in the team selection process, as opposed to wholly succumbing to the omniscient wisdom of their new robo-coach.
Where automated decision making and/or profiling does occur, the fact that a player/athlete’s personal data is being used for such purposes must be disclosed to them, together with information about the ‘logic’ involved and the intended results of processing. There are also higher levels of protection afforded under the GDPR where “special” categories of data are being processed by AI (such as ‘biometric’ or ‘health’ data collected by wearable tech). Special data can only be collected, processed and stored anonymously, or else after obtaining the data subject’s explicit consent to the processing.
Much of the ongoing legal debate in this area revolves around ownership and access to the data. If a club is using a particular technology to collect large quantities of physiological information on its players, who owns that data? Is it the club itself; the player (as the data subject); the league or sporting governing body (if the device is part of a wider initiative); or perhaps the company providing the technology? What are the specific rights and obligations on each of these stakeholders when they come into contact with the data? AI companies should be wary of a business model that relies heavily on the collection and monetisation of personal data whilst these issues remain unclear.
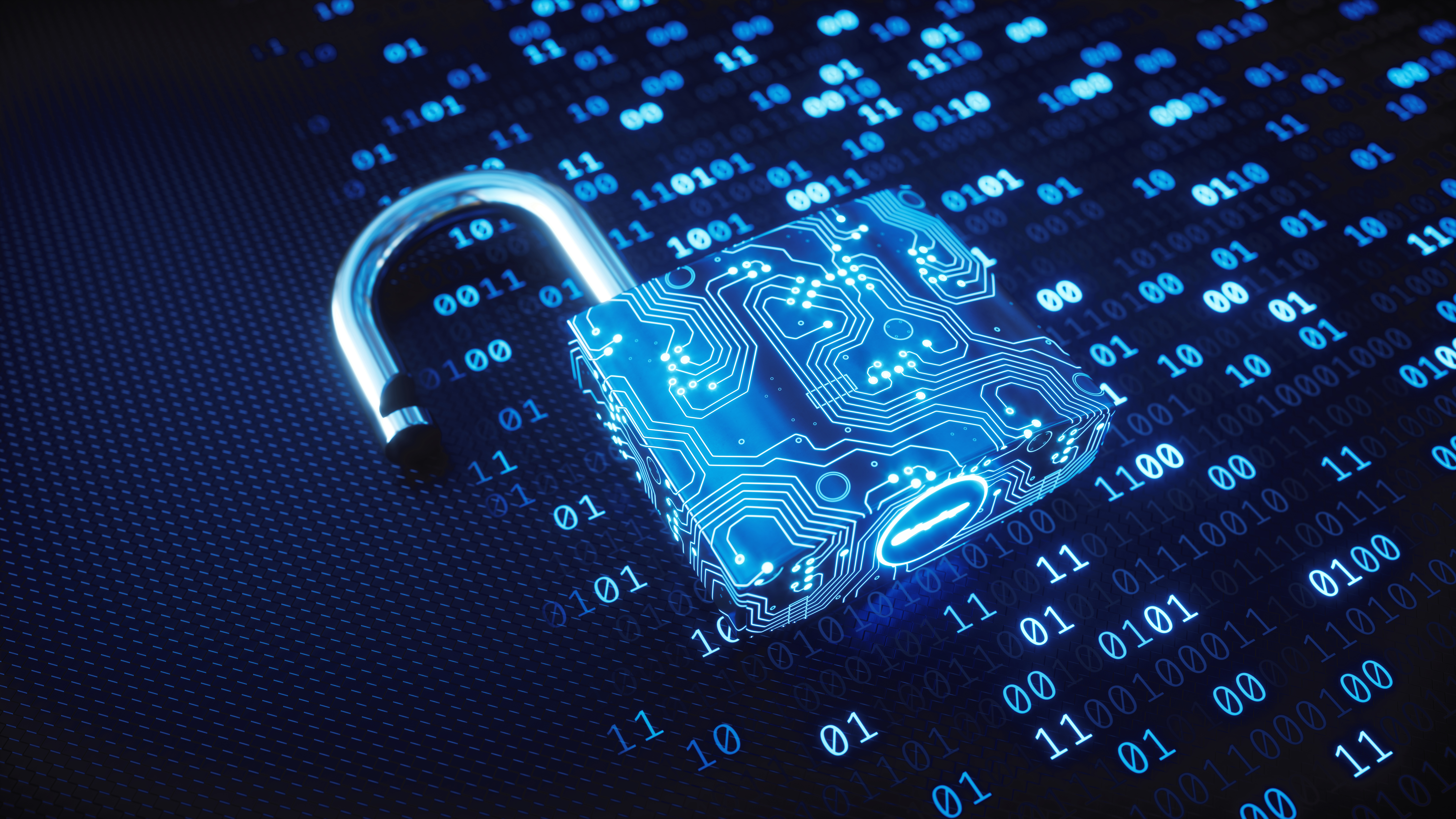
Sporting Regulations
Finally, it should be noted that various leagues and governing bodies across sport have issued regulations on the use and adoption of wearable tech in recent years.
Schedule 3 of the World Rugby Handbook, for example, sets out a number of mandatory requirements around the use of ‘player monitoring devices’, in areas such as ergonomics, construction, size, weight, and shape, and performance requirements. The specifications are made “without seeking to compromise the form or appeal of the game and with the overall goal being to promote player welfare and reduce the risks of injuries to Players as far as practicable.”
FIFA, on the other hand, dictates that where electronic performance and tracking systems (EPTS) are used in matches played in an official competition, they must contain certain insignia, cannot be dangerous, and any information transmitted from the devices/systems is not permitted to be received or used in the match area during a live game.
Conclusion
Artificial intelligence continues to strengthen its foothold in the sports industry. These smart solutions are driving exciting developments for fans in how sport is being analysed and broadcast; offering clubs new and innovative methods to gain competitive advantage; broadening reach for brands and sponsors; and forging new ground in a lucrative market for ambitious software companies.
But with seemingly unfettered growth comes fresh uncertainties for AI. Regulators are scrambling to keep up with the continually shifting technological goalposts, and key legal and ethical questions concerning the data collected remain unanswered. AI technology may be marked more tightly in years to come.
For now, let’s adopt the stance of Joey Barton before his fateful first philosophy lecture, as he tweeted: “Eyes peeled, ears open, brain engaged...”
Related items
Related sectors
Related services
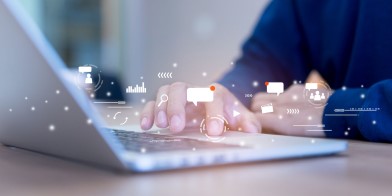
The Esports Gold Rush – What Do ‘Traditional Sports’ Need to Consider?
29 November 2019Everybody wants a piece of the esports pie, and the International Cycling Union (ICU) is the latest to make its move. The world governing body of cycling has teamed up with Zwift - the innovative online platform which allows riders to compete against each other in a virtual world - to host the world’s very first ‘cycling esport world championships’ in 2020.